7 Data Challenges in the Life Sciences
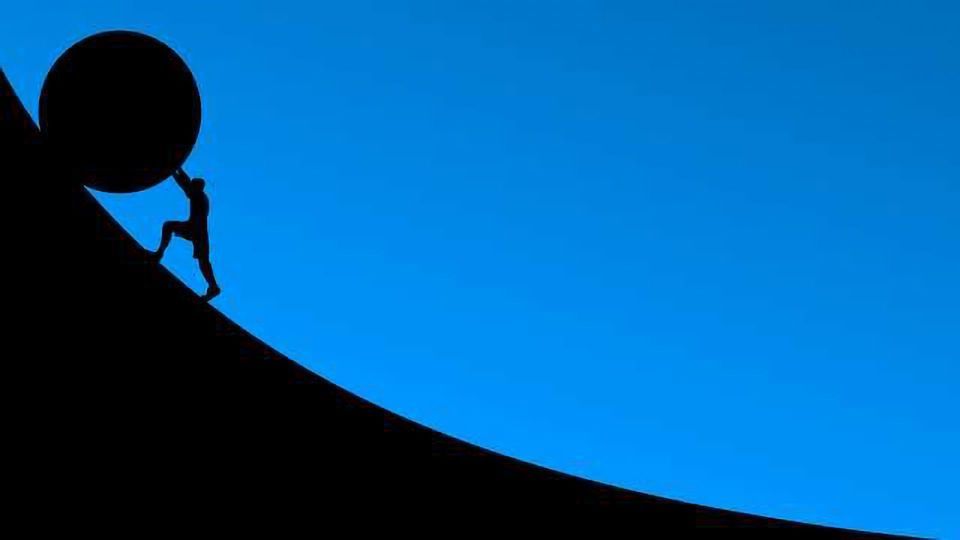
Data analysis and storage is increasingly becoming a major concern for labs across the Life Sciences. The time, cost, and complexity of data management have overtaken the cost and speed of data generation as the primary bottlenecks. All of which pose significant challenges for the scientists whose job it is to make sense of it all. Here we bring together 7 of the biggest data challenges faced by scientists right now.
Storing it all up
Modern lab equipment produces orders of magnitudes more data than cutting-edge systems from only a few years ago. From sequencing data to chemical structure information, there’s an ever-increasing stream of data intensive instruments, methods, applications and regulatory requirements.
Consider this, the amount of data be generated by genomics research per day is doubling every 7 months.1 This raw data requires expensive high-end computing to process and, introduces the challenge of data storage. Traditional, physical storage solutions are typically preferred but are expensive and bulky. Cloud storage is gaining traction but even with advances in information reduction, the cost of data-archiving can still be expensive. And, with many industries working under tight regulations it’s often not enough to simply store the data you need. Instead vast quantities of data, and meta-data must be kept securely for years to guarantee complete reproducibility.
Data standardization
Modern science depends on an integrated approach, pulling together huge teams of experts and the resources they have access to from around the world. This collaborative approach allows researchers to tackle huge projects but, also introduces huge challenges. Different instruments produce different data and different scientists record data in different ways. Without standardisation, something as simple as whether you record a patient as “Female” or simply with an “F” could make data analysis impossible. Now, scale that kind of small inconsistency up across all the data required to make something like a drug approval application – the potential for heterogeneity is beyond huge!
These issues are only compounded by a lack of standardised data formats, identifiers and loose internal data standards. We cannot forget the fact that many labs are still moving slowly into the 21st Century, transitioning their data recording systems from traditional paper-based systems into the digital world.
Availability of data
In any given project, you could have CROs, coordinators, scientists, patients and a whole host of other people generating data – all of which could be key to your research. This presents challenges when you you’re getting ready for audit, making a big decision about the direction of your research or pulling together a publication. How can you be sure that all the data you need is available?
Chances are all this data is locked up in multiple systems managed by multiple people. Simply put, your data is all over the place! Just look at this example, the 100,000 genomes project aims to sequence 100,000 human genomes in just 5 years. 13 regional health service groups are contributing, made up of thousands of health care professionals who then depend on multiple partners for sequencing, analysis and storage. From here it is easy to see how, with so many people involved, the availability of data becomes a massive challenge.
Lack of data ownership
Consider this, who is ultimately responsible for the data your company or lab produces? Many labs are faced with using the latest data they can find as there’s simply no one with the understanding to ensure the most relevant data is available. That person also needs to be confident the data from multiple sources is accurate and reliable. Left unchecked, it’s impossible to know whether your results are worth anything at all.
Ownership also naturally ties into IP and whether data should be open access. Attitudes about freely sharing data vary broadly across the scientific community. In some fields, like genomics, data sharing is completely normal. With many researchers sharing their findings in real time, accessible by anyone! Unfortunately, there are often no formal agreements within these open fields, resulting in a lack of technical infrastructure or support. Other communities struggle with data accessibility, shielding it behind pay walls or just not sharing it all. Many argue that this directly holds back scientific progress – an argument that’s not likely to go away any time soon!
Security
The scientific community faces several significant challenges in data security. With electronic data being one of the most valuable assets for any organisation, unauthorised access must be managed. Increasingly tight regulations concerning privacy laws and data traceability must also be adhered to.2 The problem is, how can you negotiate these issues whilst fostering a collaborative approach and promoting accessibility of data?
It’s certainly a challenge the scientific community needs to address. There’s been limited impact so far. But, a 2013 study has demonstrated that it is possible to re-identify research participants using easily accessible “de-identified” genomic data alongside genealogical databases and public records. 3 Scary considering that this data could be used for identity theft, blackmail, targeted health marketing and even to hike up your insurance based on the diseases you’re predisposed to!
A lack of bioinformaticians
Many argue that efforts to attract scientists into bioinformatics have been under-prioritised for years. Leading to perhaps the biggest challenge of all, finding people with the skills and experience to get results from raw data. One clear problem is the historical lack of a defined career path for a bioinformatician. The scientific community still has a long way to go in providing rewards for sharing their skills across an ever-evolving range of multidisciplinary projects. Over the last decade many institutes have launched core bioinformatics facilities to bolster their limited data expertise. But, even with these central facilities, new challenges emerge. For example, one group found that over an 18-month period 79% of techniques applied to fewer than 20% of the projects.4 Essentially, this means that most researchers came to the bioinformatics team looking for entirely customised, bespoke analysis.
The lack of clear job remit, career path and attractive rewards all seem to contribute to the ever-growing number of unfilled bioinformatics positions around the world. Looks like we need to go back to the drawing board on this one!
Sorting through the noise
So, you’ve overcome all the challenges we’ve presented so far and your data is good to go. But where to start? Within your big pile of jumbled up data you need to ascertain what’s important to your specific goals. Problem is, it’s often difficult to define what you’re looking for before you see it. So, your left to dig through your noisy data trying to spot what’s relevant. It’s also important to remember that data that’s useless to you may be mission critical for someone else. Furthermore, in many fields, experimentalists can generate new data faster than bioinformaticians can make informed predictions
Let’s look at an example, a scanning electron microscopy study on a cubic millimetre of brain tissue generates about 2000 terabytes of data.5 A scientist may only want to study one specific structure within that sample tissue. Very time consuming and plenty of room for error. Especially when a bioinformatician is called in to help as an afterthought so is not involved in experimental design.
With all that mind, it’s easy to see why big data has become one of the most pervasive issues across scientific research. And, without some serious developments in technology and even bigger changes to the way we think about data across the industry, it is one that’s only likely to get worse.
References
1. Stephens, Z. D., Lee, S. Y., Faghri, F., Campbell, R. H., Zhai, C., Efron, M. J., ... & Robinson, G. E. (2015). Big data: astronomical or genomical?. PLoS Biol, 13(7), e1002195.
2. Helvey, T., Mack, R., Avula, S., & Flook, P. (2004). Data security in life sciences research. Drug Discovery Today: BIOSILICO, 2(3), 97-103.
3. Gymrek, M., McGuire, A.L., Golan, D., Halperin, E. and Erlich, Y., 2013. Identifying personal genomes by surname inference. Science, 339(6117), pp.321-324.
4. Nature Volume 520, Issue 7546, Comment Article. Core Services: Reward Bioinformaticians. Available at http://www.nature.com/news/core-services-reward-bioinformaticians-1.17251#/unique (Accessed 23 April 2017).
5. Fuller, J. C., Khoueiry, P., Dinkel, H., Forslund, K., Stamatakis, A., Barry, J., ... & Rajput, A. M. (2013). Biggest challenges in bioinformatics. EMBO reports, 14(4), 302-304.