Visualizing Drug Targets With Ligand Express
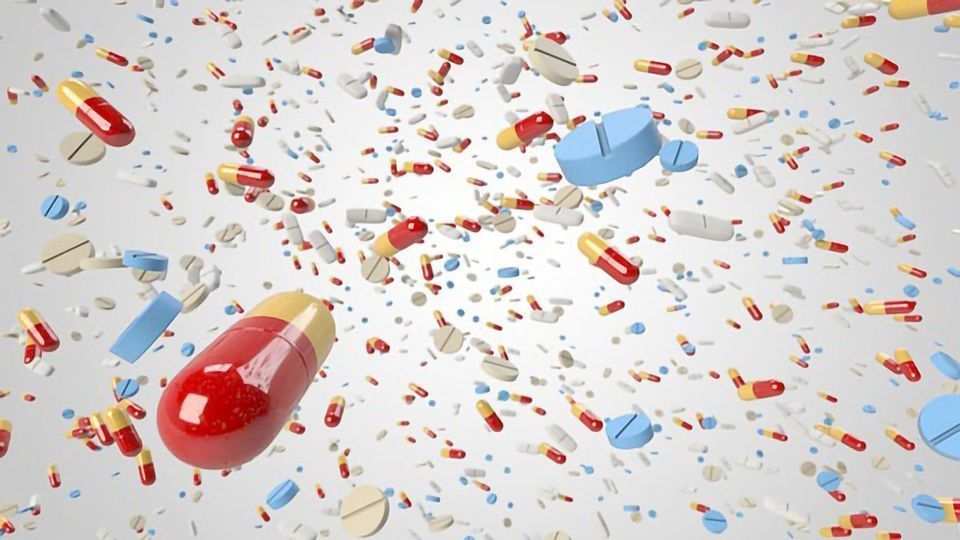
Complete the form below to unlock access to ALL audio articles.
In the development of a therapeutic pharmaceutical agent, it is crucial to understand the on- and off-target effects the molecule may have within the body. This information enables researchers to decipher the agent's mechanism of action, prioritize lead candidates, understand the side effects as well as identifying novel uses for drugs that are already marketed.
Cyclica's Ligand Express® is a cloud-based platform that screens small-molecule drugs against structurally-characterized proteins, or "proteomes" to determine polypharmacological profiles. The technology identifies protein targets by leveraging artificial intelligence and visualizes the drug's effect on these targets. Subsequently, scientists are able to identify on- and off-target interactions. We spoke with Naheed Kurji, President & CEO, and Andreas Windemuth, Chief Science Officer, from Cyclica to find out more.
Laura Lansdowne (LL): Why is it necessary to establish the exact role of a potential therapeutic target plays in a disease? Can you comment on the importance of capturing the clinical spectrum of disease?
Naheed Kurji (NK) and Andreas Windemuth (AW): In order for patients to receive the best standard of care, drugs and therapeutic agents must target the root of disease down to the molecular level. As drugs interact with dozens if not hundreds of proteins targets in the human body, drugs need to be designed for cells rather than the traditional paradigm of a single protein target. Complex diseases including cancer, nervous system diseases, and genetic diseases, require multiple drugs, each targeting a distinct protein, that work together in an efficient manner. Understanding the driving molecular mechanisms of complex diseases enables the development of efficacious drugs that target proteins or pathways directly associated with the disease. At Cyclica, we offer an end-to-end enabling platform that allows scientists to design, screen, and personalize drugs while examining multiple targets simultaneously. This comprehensive approach to drug design is critical in creating drugs that target relevant proteins in the body while avoiding adverse outcomes. We are equally focused on driving insights into structural pharmacogenomics by incorporating elements of genomic data, specifically single nucleotide variant and polymorphism data in our platform. As a result, we will be able to map physical molecular interactions responsible for differences in drug-response due to genetic variation. This is a key step in achieving precision medicine, the discipline of developing treatments for patients based on a genetic understanding of a specific disease.
LL: Why do some of the more traditional drug discovery approaches fall short?
NK and AW: Today, it takes around 13 years and costs approximately $2.65 billion to bring a drug to market, representing an 80x productivity decline over the past 50 years. According to a Shih et al. 2017 Nature Reviews publication, “The single biggest contributor to the cost of drug research and development (R&D) is the high project attrition rate. Attrition is due to various reasons, including insufficient efficacy, toxicity and strategic reasons.” A key reason for the high attrition is that traditional drug discovery is focused on designing or screening the best drug to interact with a single target. Conventional molecular modeling approaches for drug design, or screening methods like high-throughput screening (HTS) or virtual screening (VS), start with a single biological target and then screen a library of drugs to identify those which interact with that target. While these approaches are useful, they only take scientists so far. It is now well understood that drugs interact with many proteins in the body, with some studies estimating anywhere between 30 and 300 individual interactions, many of which are not known up front. While the narrow approach provides scientists with a shorter list of putative drug candidates that interact with known targets, it does not provide them with the broader picture of what else a drug is doing in the body, i.e. the other proteins a drug is interacting which is referred to as its polypharmacology. Experimental approaches to uncover polypharmacology (eg: CETSA or other Mass Spec approaches) are restricted to available targets for wet-lab assays, and therefore not able to scale or uncover previously unknown information. This scientific and technological gap is where Cyclica saw an opportunity and is what led to the formation of the company and the development of Ligand Express®. Recent advancements in applying deep learning to conventional VS through convolutional neural networks (CNN) have emerged as noted by Ragoza et al. in 2017, who present on open source tool but also raise substantial concerns over the approach. Ragoza et al. found that a CNN enhanced VS technology that is trained on publicly available data struggles to deliver value because the available data are too limited. In any case, none of the available CNN based methods (Ragoza et al., 2017, Wallach et al., 2015) address polypharmacology.
Overall, pharmaceutical companies need a faster and cheaper way to discover and develop safer and more effective medicines for patients with unmet needs. We firmly believe that to achieve this, we need to not only enhance existing methods, but also innovate novel, end-to-end enabling technologies that drive value at multiple stages of the discovery pipeline. Importantly, these technologies must augment internal workflows and enhance how scientists make faster and cheaper “go” and “no-go” decisions.
LL: What are off-target interactions, and what impact can these have on the drug discovery and development process?
NK and AW: Off-target interactions are essentially biology-altering interactions with proteins beyond a drug’s intended therapeutic target, which often result in unanticipated and undesirable side effects. The traditional target-centric model as described above does not account for these off-target interactions that cause an increase in cost, time, and effort. This phenomenon where drugs may have hundreds of off-target interactions is called polypharmacology. A toxic side effect found late in the drug discovery pathway (after hundreds of millions of dollars have been spent), can result in huge setbacks and an increased risk for patients. An approach focused on polypharmacology is a critical shift towards designing drugs in a holistic manner by looking at the cell as a whole, not just a single protein. A growing body of literature has demonstrated the increasing significance of polypharmacology given the complexity of disease and the unwanted interactions that can cause adverse effects. At Cyclica we have a computational approach and a polypharmacology philosophy, which together delivers insights into a molecule’s mechanism of action, explores new targets for drug programs, repurposes existing drugs for new indications (i.e. new targets), and designs multi-targeted drugs that address the spectrum of disease at the molecular level. Figure 1: Drugs are designed for one target (green), but once introduced in the body result in many unanticipated, or off-target interactions (red) that may cause side effects. This phenomenon is called polypharmacology.
Molly Campbell (MC): How are artificial intelligence utilized to visualize the predicted drug-protein interactome?
NK and AW: At Cyclica we firmly believe artificial intelligence (AI) plays a critical role in the future of drug discovery, accelerating R&D innovation and increasing productivity. AI will present scientists with new information so they can enhance their level of performance and process information at a faster rate. However, AI will not be the silver bullet, and it certainly will not replace scientists. We believe it is the combination of biophysics and AI that will revolutionize drug discovery and shift the paradigm from classic single-target interactions to holistic cell-based drug design. AI is most useful when it enhances biophysics and chemoinformatics approaches. Our cloud-based platform Ligand Express is used by scientists in pharmaceutical companies to support their efforts and provide an integrated workflow. Through MatchMaker™, a structure-based and AI-augmented technology that powers Ligand Express, scientists can uncover how a drug interacts with a target at a molecular level, and with AI, further understand this data to make predictions on modulatory effects. Cyclica’s AI is supported by drug-protein interaction information, coupled with systems biology, that links proteins to function, disease, and other small molecules.
MC: How have advances in proteomics supported the development of Cyclica’s technology?
NK and AW: Proteome screening presents certain challenges, most of which is its computational complexity. The past few years, however, have presented an increase in computational power and machine learning algorithms which largely rely on existing knowledge of drug binding interactions and provide insights into a marginal percent of proteins. At Cyclica, we have addressed this problem by bringing together computational chemistry and big data to focus on proteome-wide screening. Our proprietary Ligand Express MatchMaker™ technology assesses the likelihood of an interaction between a small molecule and thousands of protein structures. Previously, a proteome screening approach was considered problematic due to a lack of structural data on proteins. Now, 80% of the proteome has structural data associated with it and even more is being generated at an exponential rate. With this advancement in data, we can screen small molecules against all known structurally characterized human proteins thereby providing a comprehensive, holistic understanding of a drug’s effects in the body, and both on- and off-target interactions across the entire proteome.
MC: How is Cyclica helping scientists advance the field of drug discovery?
NK and AW: Our platform Ligand Express, a cloud-based, integrated, end-to-end enabling platform, is used by scientists in pharmaceutical companies to accelerate their drug discovery efforts. The vision for Ligand Express is to be an integral utility platform in the pharma R&D value chain and support the work of scientists in a collaborative manner. We believe it is this integrated workflow that will enable scientists, biochemists, medical chemists, and the like, to make efficient decisions regarding drug discovery. Together with scientists, we believe our technologies can be leveraged to understand the plethora of existing data. For instance, we have built a collection of predictive ADMET models using proprietary AI methodology to facilitate the translation of small molecules into drugs. This technology has demonstrated enhance predictive accuracy for generating ADMET compared to traditional classifiers (read more here). As such, Cyclica’s platform, Ligand Express, and predictive ADMET technology helps scientists make informed decisions thereby reducing downstream costs and potential risks.
Naheed Kurji and Andreas Windemuth were speaking to Laura Elizabeth Lansdowne and Molly Campbell, Science Writers at Technology Networks.